Last month, I had the fantastic opportunity to attend the Big Data and AI Conference 2023. As an avid fan of cutting-edge technology and data-driven insights, this event was a great opportunity. My main reasons for attending were twofold: to gain a North American perspective on the evolving landscape of Big Data and AI and to connect with like-minded individuals and businesses. So, I wanted to reflect on what I learned whilst collaborating with experts, innovators, and fellow data enthusiasts. Below are three key takeaways that left a lasting impression.
Retrieval Augmented Generation in Large Language Models
One of the most exciting discussions at the conference was the applications of retrieval augmented generation (RAG) techniques in LLMs. This innovative approach combines deep learning with information retrieval, enabling LLMs to generate contextually rich and accurate responses. In a typical conversational AI bot, such as GPT-3, the model can generate responses based on patterns learned from vast amounts of text data. However, they often struggle with generating contextually accurate and information-rich responses. By implementing new RAG techniques, LLMs can now tap into external knowledge sources and retrieve relevant information for their responses. Enabling them to provide answers that are based on up-to-date, specific information.
Through the integration of machine learning algorithms, the LLM can analyse the feedback data. This allows the identification of patterns in user ratings and understand what makes a response good or bad. Based on the feedback, the LLM can be fine-tuned to generate better responses in the future. It can learn to prioritize certain sources, improve context understanding, and enhance its language generation capabilities. In a business context, by using RAG based LLMs in customer support, it can lead to more satisfying interactions. Customer service agents can now ask bots questions and receive more accurate, references, context specific answers.
See below an example from Data Robot’s RFP bot which achieves just that. In Slack, when a user asks a question, the bot will search through the documentation and provide an answer. Then references this answer, evaluates itself and then allows the user to provide an evaluation.
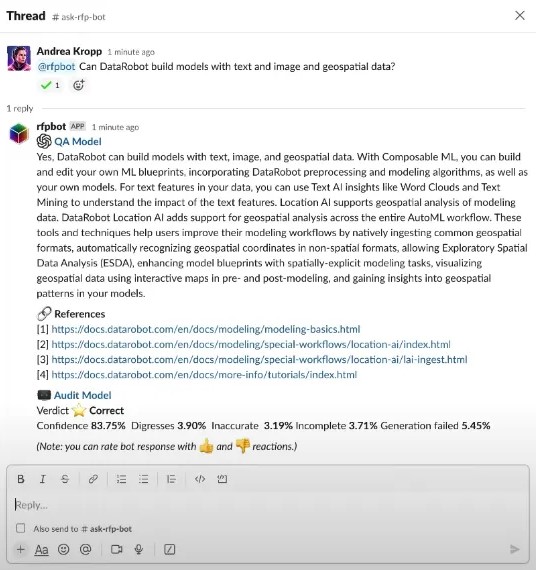
The Rise of Citizen Analysts Within Companies
Another memorable topic that emerged during the conference was the growing rise of “citizen analysts” within organisations. With the democratization of data analytics tools, the increasing availability of training resources, and the shortage of data experts, employees from various departments are taking ownership of data analysis. This shift empowers individuals to make data-driven decisions, drive innovation, and foster a culture of continuous improvement. The rise of citizen analysts also promotes cross-functional collaboration and a deeper understanding of business insights. It’s a trend that underlines the importance of data literacy for professionals across all industries.
The benefits of promoting citizen analysts within businesses include:
- Citizen analysts can make decisions, ad-hoc analyses and generate insights quicker than traditional data science teams, leading to more agile decision making.
- Citizen analysts can be more cost-effective than hiring expensive, dedicated data scientists.
- Citizen analysts often have a deep understanding of their industry and domain, enhancing the relevance of their analyses.
- Encouraging employees to become citizen analysts can boost engagement and job satisfaction. As it empowers them to contribute to data-driven improvements.
However, researchers warn of multiple hurdles facing citizen analysts, which include:
- Citizen analysts may face challenges related to data quality, availability, and accessibility. Ensuring data is accurate, up-to-date, and well-organized can be a significant hurdle.
- Citizen analysts may have analytical skills, but they may lack in-depth technical expertise in data science tools and programming languages, limiting their capabilities.
- Analysing sensitive data requires an understanding of data ethics and privacy regulations. Citizen analysts must be trained to handle data responsibly.
- Integrating citizen analysts’ work with existing IT infrastructure and data governance practices can be challenging.
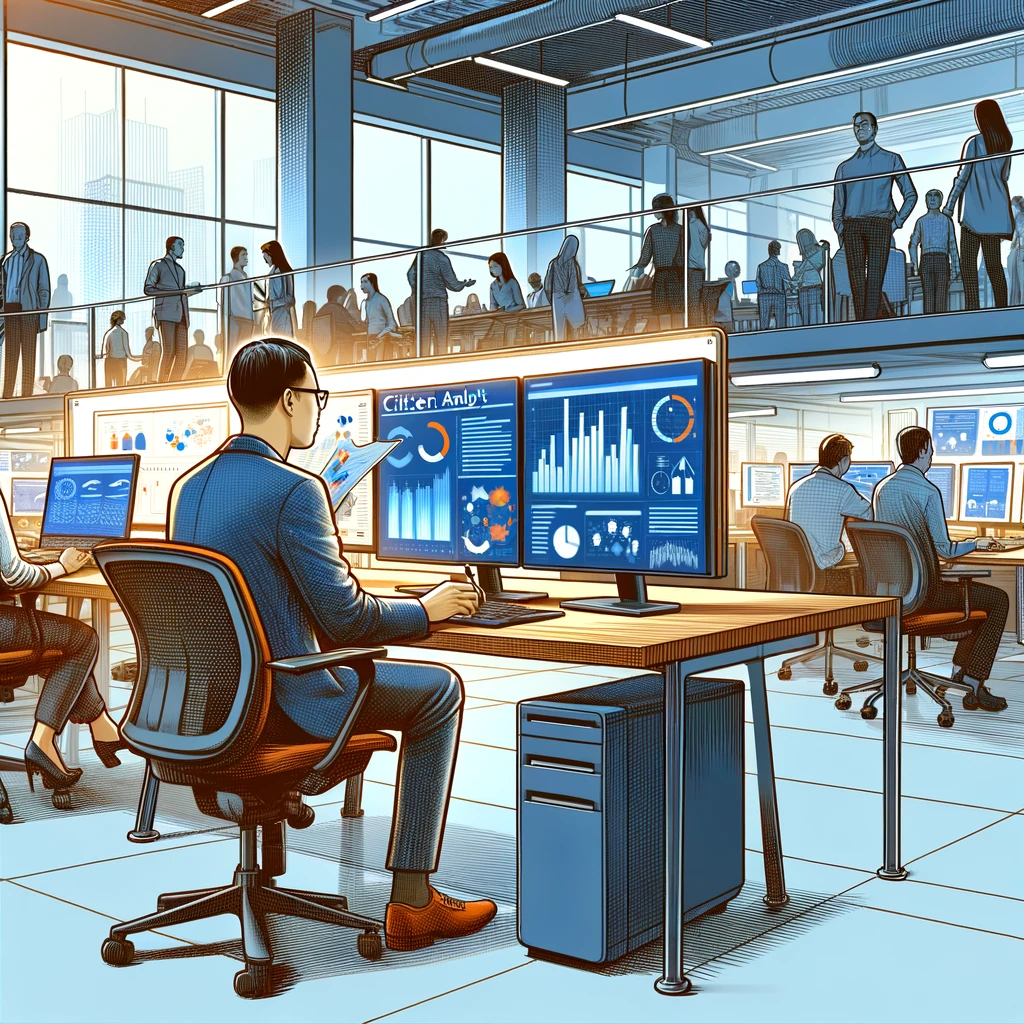
Data Clean Rooms
Nowadays, data privacy and security are paramount, so organisations are now exploring new methods to share and analyse data without compromising individual privacy. Data clean rooms provide a controlled environment where multiple parties can collaborate on data analysis projects while ensuring compliance with privacy regulations. This approach has the potential to unlock valuable insights from sensitive data sources, such as healthcare records or customer behaviour data, while respecting privacy rights. Data rooms facilitate the agregation and storage of data from different sources, including customer data, healthcare records, or financial information. By anonymising the data before entering the clean room it removes sensitive data that could identify individuals. To safeguard the data, access controls and encryption measures are put in place .
Data analysts and researchers can then access the aggregated and anonymized data within the clean room to perform various analyses, such as market research. Collaboration tools and platforms facilitate teamwork while ensuring data privacy. Every action within the data clean room is logged and audited to ensure transparency and accountability. Clean rooms enable organizations to collaborate on data analysis projects with partners, suppliers, or research institutions while maintaining data privacy and confidentiality. Businesses can extract valuable insights from large datasets, including merging data from multiple sources for a more comprehensive understanding of trends and patterns.
A data clean room facilitator plays a crucial role in managing and securely keeping data legally compliant. The facilitator establishes and enforces data governance policies and procedures to ensure that data is handled in accordance with legal and ethical standards. They manage user access to the clean room, ensuring that only authorized individuals can enter and work with the data.
Overall Experience
My trip to the Big Data and AI Conference 2023 in Toronto was an eye-opening experience filled with knowledge sharing, networking, collaboration. The field of Big Data and AI continues to evolve at a rapid pace, and staying up to date with the latest trends and innovations is essential. I’m grateful for the opportunity to have gained a North American perspective and to have connected with incredible individuals. The future of Big Data and AI is exciting, and I can’t wait to see where the three topics discussed take us next.